The promises of advanced analytics are inherently appealing to businesses: make real-time, data-informed decisions, optimize and automate processes, and monetize data in new ways. In the first installment of a three-part blog series, we’ll zero in on the first of five key success factors for establishing an advanced analytics capability: building analytics teams with strong leadership and alignment to business value.
Forrester notes that companies performing solid advanced analytics grow 30 percent faster than their competitors, and are expecting $1.8B in extra growth purely from analytic strength. Often though, the challenge of advanced analytics can seem overwhelming. Merging data scientists with business teams and existing IT groups to deliver on this promise poses significant challenges. We know that 92 percent of companies have “increased investment in Data & Artificial Intelligence,” but we also know that from these same companies, 77 percent report that integrating these initiatives is a “major challenge.”
To overcome these challenges and unlock the promises of advanced analytics, most organizations take their first strides through preexisting business intelligence (BI) capabilities. And for good reason: these teams have similarities such as shared skillsets, required inroads with the business, and familiarity with the flow of data through the organization. Some BI resources may even have direct advanced analytics skills to immediately prototype work. Even though these teams are not likely ready to operationalize the results, their first attempts are often enough to demonstrate quick-wins and business value that build the case for future team investment.
So where to go next? How do you take these humble beginnings and mature them over time, simultaneously progressing the multitude of areas required to establish full lifecycle delivery of advanced analytics?
Professionalizing advanced analytics, ultimately, is a business transformation process. It is not just a technical exercise: Change management, team development, tools, processes, and integration into business goals are all foundational to this effort. We’ve identified five critical success factors to establishing a proper advanced analytics capability:
- Ensure analytics teams have strong leadership and alignment to business value
- Develop an analytic organization and culture through proper role identification, career development paths, and recruiting
- Establish modern tools that fit into existing technical infrastructure
- Have a well-defined development methodology to lead from idea to prototype to production deployment
- Operationalize models for proper business value
Today, we’ll unpack the first key success factor: Ensure analytics teams have strong leadership and alignment to business value
Data science can be applied to almost any problem, but just because a problem is compelling doesn’t mean it promises ROI. Empowering a strong leader over an analytics group that can confidently set proper scope is one of the first goals that an advanced analytics transformation should target. To achieve this, it’s crucial that the leader embody two core skillsets, underpinned by an ability to balance the two:
- A good working relationship with business teams
- An understanding of how data science is performed
1. Working relationship with business teams
Since data science initiatives live or die by the buy-in provided by the business teams that own the results, the advanced analytics leader must earn respect by truly understanding business needs. It’s crucial for this leader to draw out the early adopters and build the base of internal supporters. By establishing early wins, this business sponsor group will become an advocate and help promote future projects. These early wins need not be major initiatives: supporting small tactical decisions or providing decision support for simple processes can reduce the mental load of knowledge workers and allow them to do their jobs more efficiently and confidently.
2. An understanding of how data science is performed
Second, the leader must be well-versed in the components of end-to-end delivery of advanced analytics—including knowledge of statistics and machine learning and the inherit limitations, toolsets, delivery methodology, and IT systems, etc. This understanding is critical to navigate the path from proof-of-concept to operationalized results and, ultimately, to deliver on the promise of advanced analytics. Just as the leader must be credible with the business, they must be credible with the analytics team. A leader who supports his or her team and represents them well can inspire confidence and trust in the development team. For example, if an analyst presents preliminary results, it is crucial that his or her manager understands the context under which the model was developed and any constraints that may apply—and crucially not overpromise the results based on initially positive indications. Similarly, if the analyst comes back with bad news, an experienced leader can help redirect to other potential analytical options or business data sources.
In the next part of our series, we’ll look at the role of the organization and technology in mobilizing advanced analytics capabilities.
Related Content
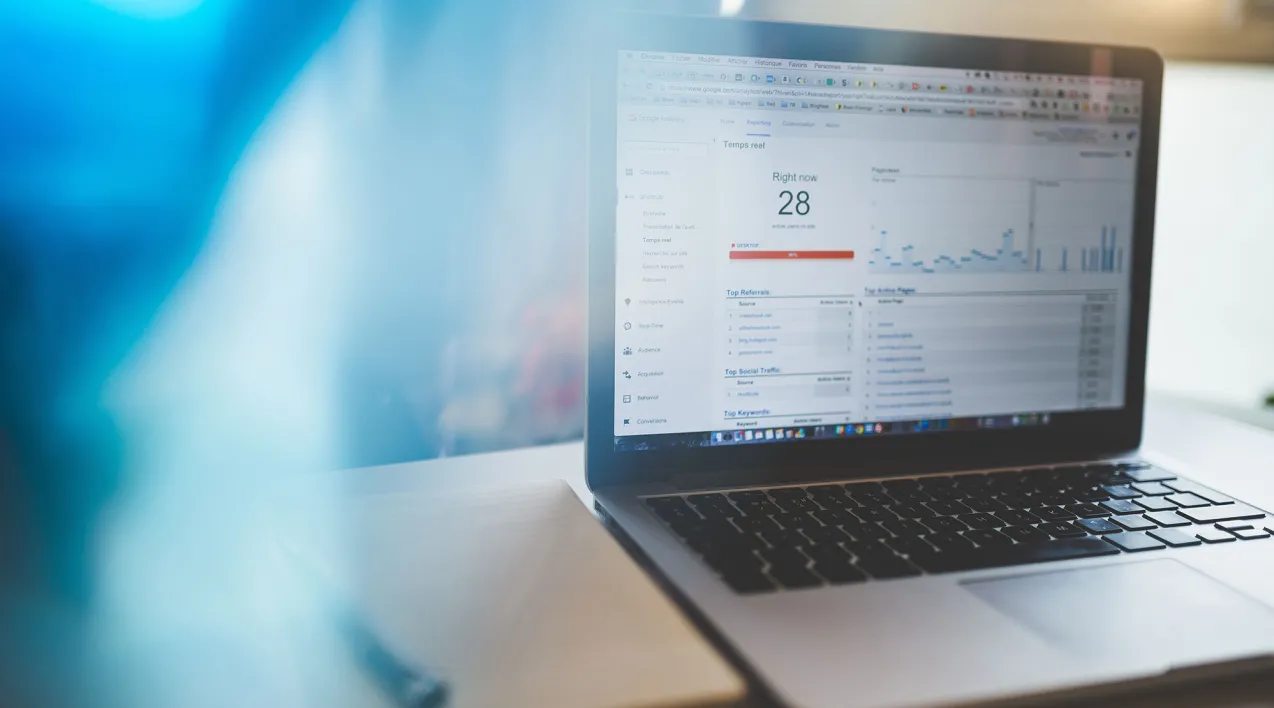
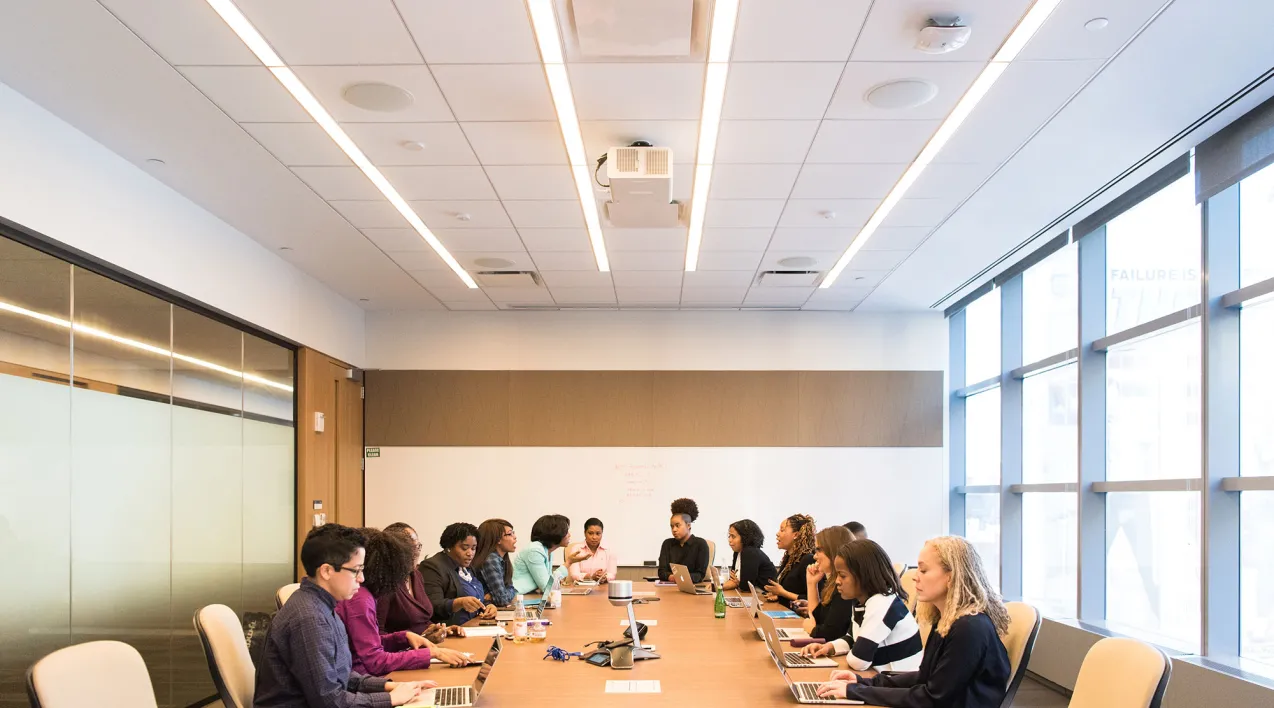